We’ve established an approach called Fair Rate which is based on equally splitting the additional profits generated by working with MT with all parties involved.
The deal is simple: we assure a fair arrangement where we decrease the MT payable rate proportional to the improvement in MT quality, ensuring translators’ earnings increase.
For example, Hanna currently translates an average of 600 words per hour from American English to Italian and earns 24 euro per hour. If MT improvements in the near future help her translate 50% more words per hour, she will earn 25% more: in this case, 30 euro for 900 words in an hour of work.
Here’s a projection of translators' hourly earnings from now until 2030 considering a stable per word rate, based on the data we've collected on MT quality improvement since 2011.
Let’s now delve deeper into the reasons behind our payable rates.
How We Calculate The Fair Rate for MT Suggestions
We initially established a metric to assess the cognitive effort required by translators when post-editing a document. This metric, Time To Edit (TTE), indicates the time professional translators need to check and correct MT-suggested translations. It refers to the time needed to edit a single word and is calculated as the total time a translator spends post-editing a text segment divided by the number of words in that segment.
TTE has proven to be the most reliable measure of MT quality, as it is defined by the average time taken to comprehend the meaning of a text segment, reflect upon it, and amend the machine-translated output in a real working scenario.
With this, we’ve established TTE as the parameter to define a fair post-editing payable rate.
To measure TTE accurately, we developed a computer-assisted translation (CAT) tool called Matecat, which is free to use and integrates our adaptive machine translation. The project was funded by the European Union and developed in collaboration with Fondazione Bruno Kessler, the University of Edinburgh, and the Université du Maine. In 2014, it was released as open-source software. Eight years later, we were astounded by the results Matecat revealed. The continuous decline in TTE shows a strikingly linear pattern, indicating that the quality of MT is progressively approaching a state where translations are increasingly correct from an accuracy and grammatical point of view.
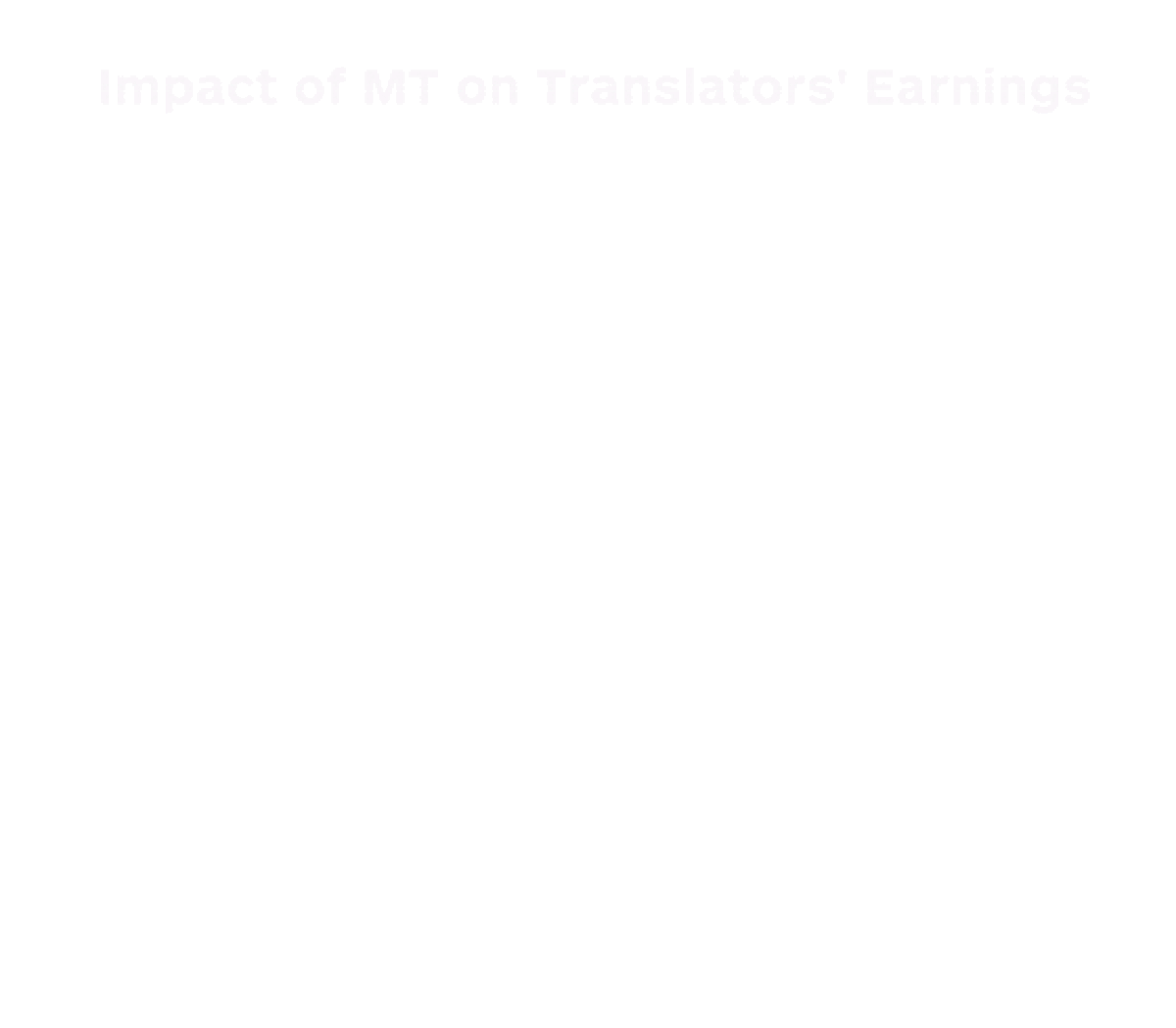
When we analyze a graph that represents the relationship between TTE data and words per hour processed by translators, we can see that over the years, translators' average speed has increased considerably when working with MT. This improvement has increased translators' per-day capacity, and consequently their earnings, allowing us to adjust the payable rate while ensuring mutual satisfaction.